Computational Modeling of Signaling Networks
Participants: Allen, Hiltbold, John, Loeser, Muday, Norris, Poole, Turkett
Questions: This group brings together biologists who generate original data sets and computer scientists and mathematicians who build models to find patterns and relationships in these data sets. Investigators focus on the computational modeling of networks of molecules that facilitate communication within and between cells.
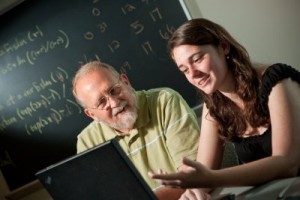
The group is currently working to understand the difference between two different types of mathematical models that can be constructed (next-state and co-temporal network models) as they relate to biological systems. These researchers are developing techniques to computationally verify the associated algorithms with real biological data, in order to move beyond theoretical data that is often used in the associated literature.
Technology: This group has developed and implemented three different algorithms for the computational modeling of molecular communication. Broadly speaking, the three algorithms can be described as discrete Bayesian, continuous Bayesian and computational algebra. The first two approaches (the “Bayesian” approaches) employ probability-based statistical techniques to compute the likelihood that a given hypothesized network model fits a set of biological data. A computational algebra approach also searches to find polynomial functions, using the measurements of gene/protein entities as values for variables, to generate the observed data using both next-state and co-temporal approaches.
A number of software tools have been developed, many as the result of Masters thesis work of graduate students in the Departments of Mathematics and Computer Science. This work includes methods for employing robust clustering on replicate microarray data sets and methods for searching across known network models to find gene/protein subnetworks exhibiting significant changes in gene expression (as observed on a microarray). This second tool plays a large role in aiding verification of the modeling tools being developed, as it allows for biologically interesting known networks to be extracted from public and commercial databases. This group requires computational resources, including both hardware and software. These modeling algorithms have been designed to be run on multi-processor computers (such as WFU’s DEAC computing cluster) and generate significant amounts of output.
Emphasis Group Activities: This group has a very successful Computational Modeling of Signaling Networks weekly group meeting that has been a fruitful forum for collaboration and communication. As this structure is working well, it will continue as a model for the other groups within the center and will grow with additional participation beyond its current membership. Activities of this subgroup are organized by William Turkett.
Implications: In the July 1, 2005 issue of Science, Elizabeth Pennisi described one of the top twenty-five unanswered questions in science in her article How will Big Pictures Emerge From a Sea of Biological Data?. The problem is that there is a great deal of biological and cellular information available, but no proven techniques for organizing this data into appropriate network models. Verification of these algorithms is an important open question, with great potential to inform biologists about meaning in their data sets, which cannot be addressed without powerful computational and modeling approaches.